Machine Learning Applications & Real-World Impacts
Machine Learning is transforming industries like healthcare, finance, and retail by automating complex tasks and improving decision-making. Discover the fundamentals, key applications, challenges, and exciting future directions of machine learning in Part Two of our 'AI Demystified' blog series.
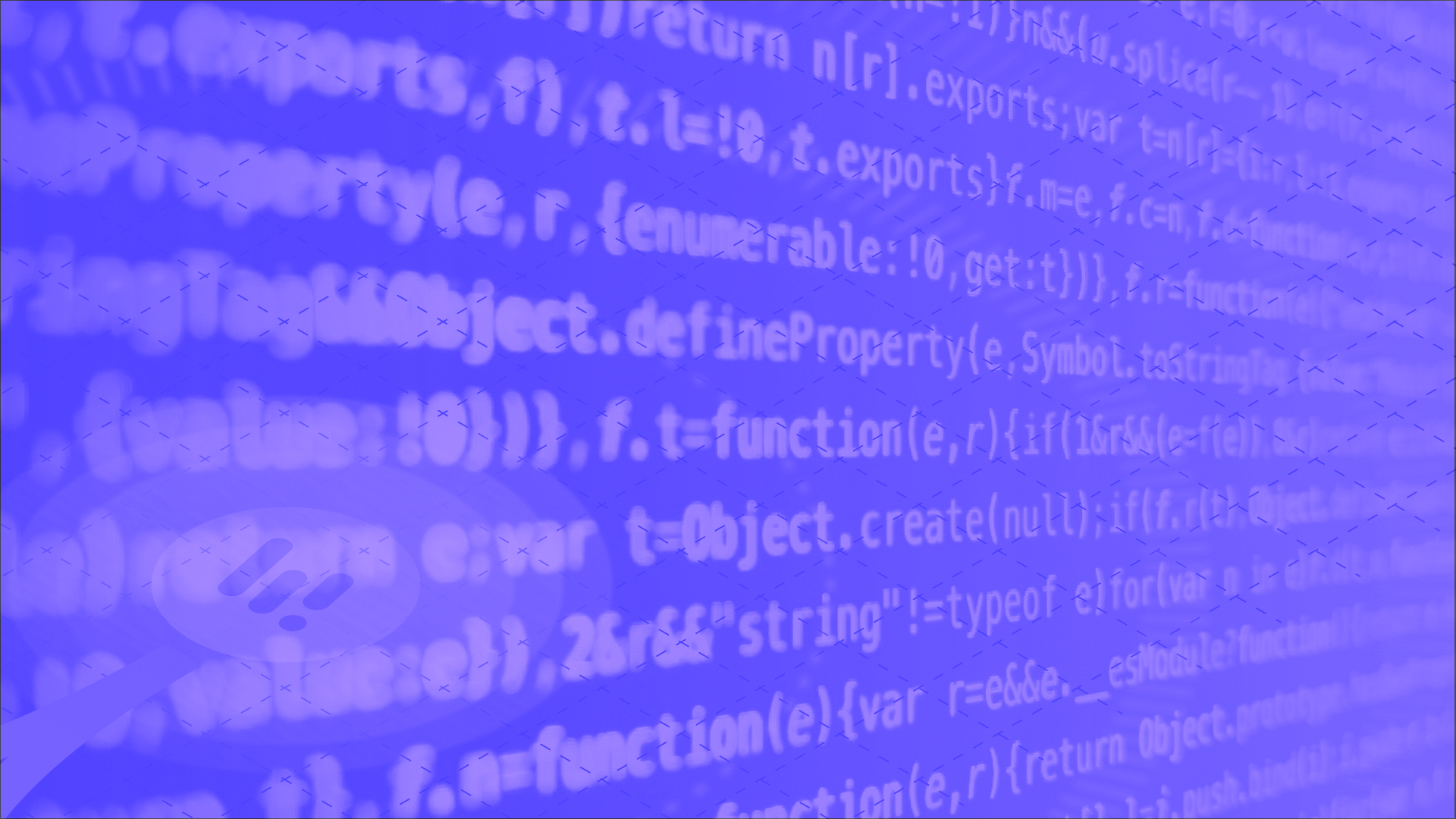
Welcome back to the "AI Demystified" blog series! This is Part Two, where we're diving into the fascinating world of Machine Learning (ML). Missed the first part? Check it out here. If you've ever wondered how Netflix knows what you want to watch next, how machine learning in healthcare helps with predictive diagnostics, or how your bank uses AI for fraud detection—it's all thanks to ML. Today, we'll explore the fundamentals of machine learning, its real-world applications across industries, the challenges it faces, and where it's headed. Let's keep it relaxed, informative, and yes, a little fun—no intimidating jargon here!
Machine learning has become a cornerstone of modern technology, driving innovation across various sectors and reshaping how we interact with data. As a subset of artificial intelligence (AI), machine learning focuses on building systems that can learn from data, enabling them to make predictions, automate complex tasks, and enhance decision-making processes. In this blog, we will explore the fundamentals of machine learning, its applications in different industries, the challenges it faces, and its future potential.
Understanding Machine Learning Fundamentals
Definition and Importance
Machine learning is a field of AI that involves creating algorithms and models that allow computers to learn from data. Unlike traditional programming, where rules are explicitly defined, ML systems identify patterns and relationships within data to make predictions and decisions. This capability is essential for automating complex tasks and providing insights in scenarios where traditional approaches fall short.
Types of Machine Learning
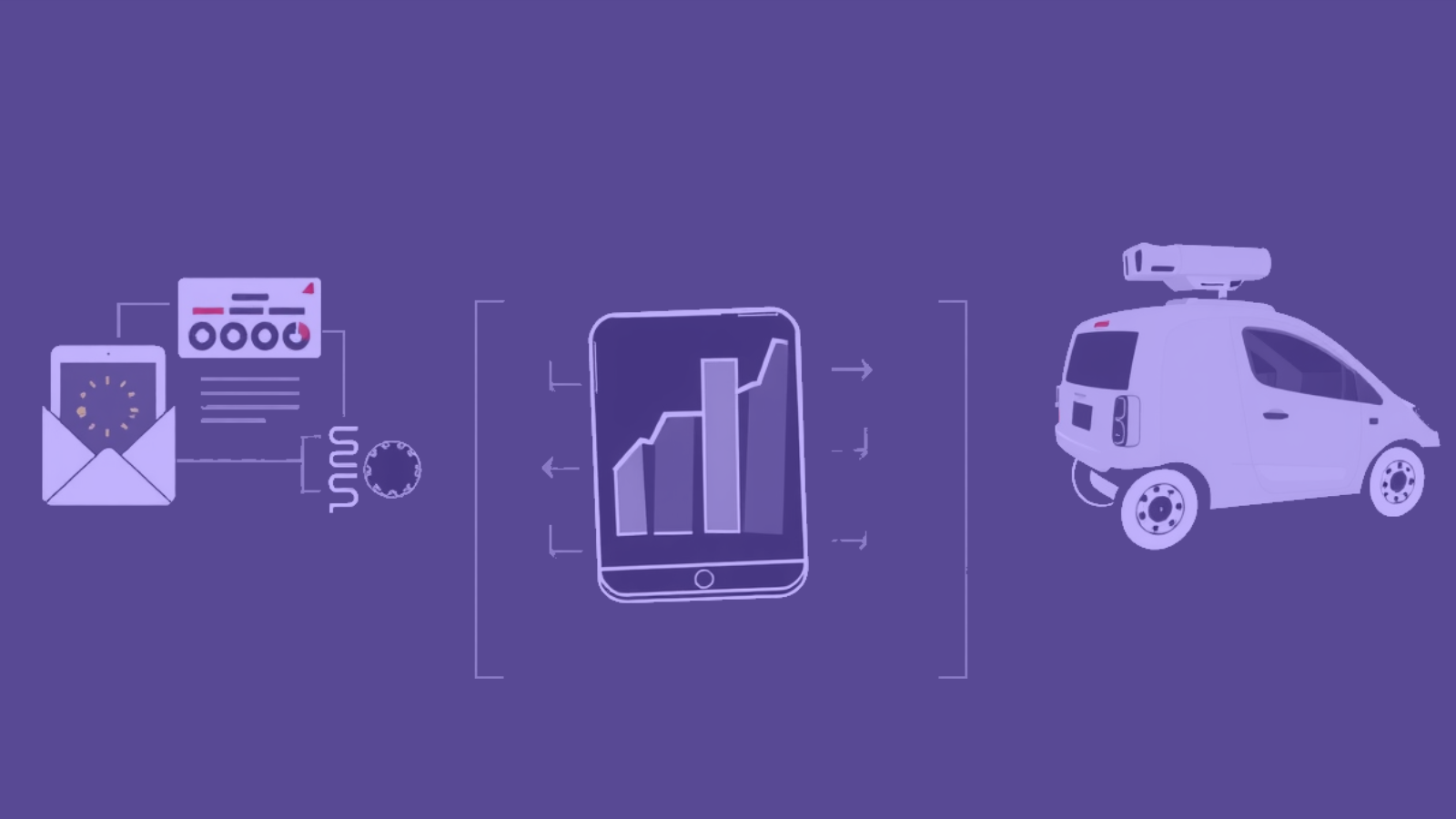
Supervised Learning
In supervised learning, algorithms are trained on labeled data, where the correct output is provided for each input example. The goal is to learn a mapping from inputs to outputs, enabling the model to make accurate predictions on new, unseen data.
- Example: Email Spam Detection
Supervised learning algorithms are used to classify emails as spam or not spam based on features like keywords, sender information, and email structure. By learning from labeled examples, the model can identify patterns associated with spam emails and effectively filter them out.
Unsupervised Learning
Unsupervised learning involves training algorithms on unlabeled data to identify underlying patterns or structures. These models aim to group similar data points or detect anomalies without predefined categories.
- Example: Customer Segmentation in Marketing
Retailers use unsupervised learning algorithms to segment customers based on purchasing behavior, preferences, and demographics. This segmentation allows businesses to tailor marketing strategies and offers to specific customer groups, increasing engagement and sales.
Reinforcement Learning
Reinforcement learning involves training agents to make decisions by interacting with their environment and receiving feedback in the form of rewards or penalties. The agent learns to maximize cumulative rewards over time by exploring different actions and their outcomes.
- Example: Robotics and Autonomous Vehicles
Reinforcement learning is applied in robotics to teach machines how to navigate and perform tasks in dynamic environments. Autonomous vehicles, for instance, use reinforcement learning to learn driving behaviors and make real-time decisions based on road conditions, traffic, and obstacles.
Machine Learning Applications
Machine learning has permeated various industries, driving innovation and improving operational efficiency. Let's explore some of its key applications:
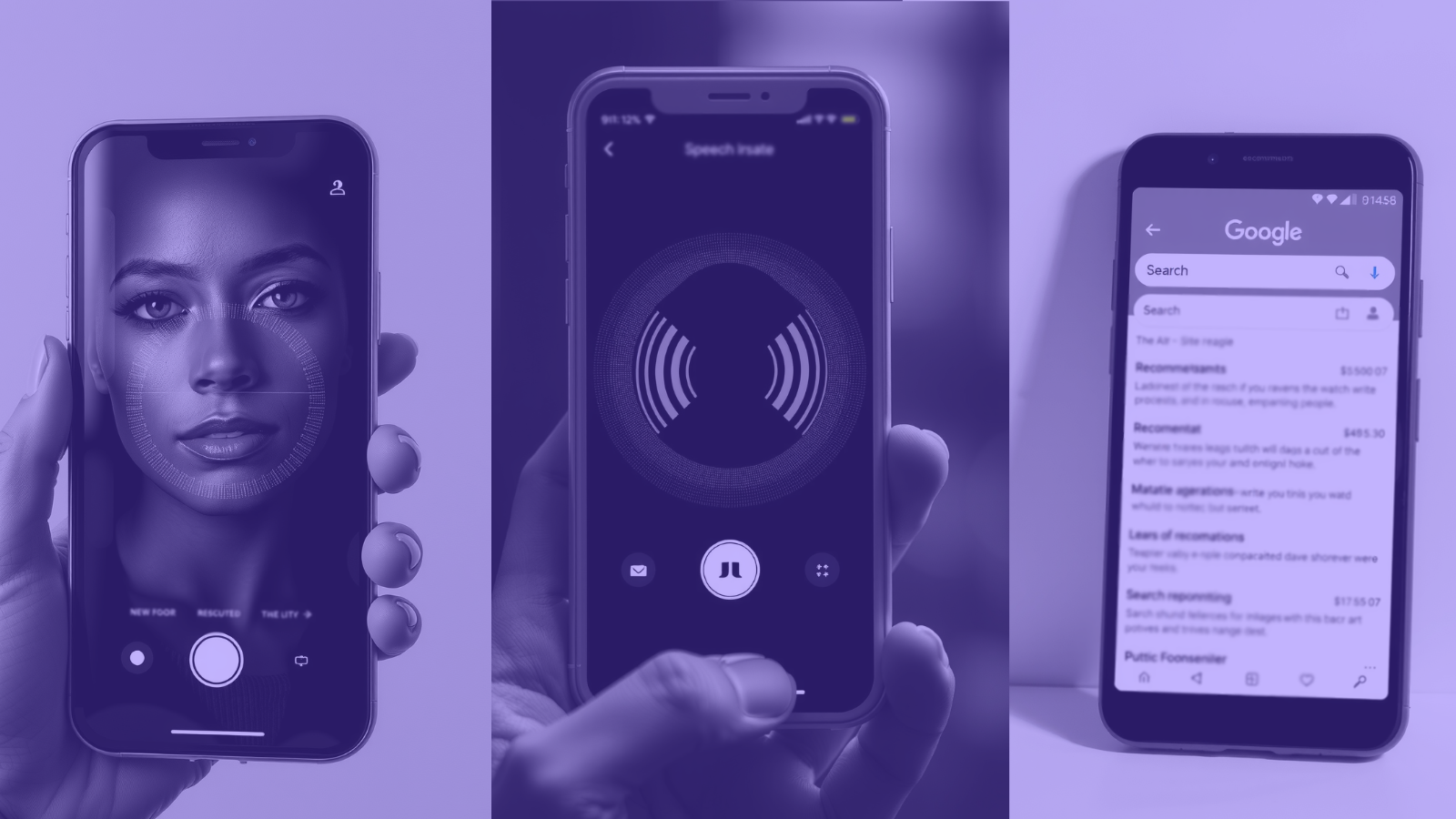
Industry-Specific Uses
Healthcare: Predictive Diagnostics and Personalized Medicine
Machine learning is transforming healthcare by enabling predictive diagnostics and personalized treatment plans. Algorithms analyze medical data, including patient records and imaging, to identify early signs of diseases and recommend personalized interventions.
Example: Predictive DiagnosticsMachine learning models can predict the likelihood of diseases such as diabetes or cancer by analyzing genetic, lifestyle, and clinical data. This early detection allows healthcare providers to implement preventive measures and improve patient outcomes.
Finance: Fraud Detection and Algorithmic Trading
In the finance sector, machine learning enhances security and trading strategies. ML algorithms detect fraudulent activities by identifying unusual patterns in transaction data, reducing financial losses for institutions and customers.
Example: Fraud Detection Machine learning models monitor transaction data in real-time to detect suspicious activities, such as unusual spending patterns or unauthorized access. Financial institutions use these models to prevent fraud and protect their clients' assets.
Example: Algorithmic TradingMachine learning algorithms analyze market data and trends to execute trades at optimal times. By leveraging historical data and predictive modeling, these algorithms can make split-second decisions, maximizing returns for investors.
Retail: Recommendation Systems and Inventory Management
Retailers leverage machine learning to enhance customer experiences and streamline operations. ML models analyze customer preferences and behaviors to provide personalized product recommendations, increasing customer satisfaction and sales.
Example: Recommendation SystemsOnline platforms like Netflix and Amazon use machine learning algorithms to recommend content or products based on user preferences and past interactions. These systems analyze viewing or purchasing history to suggest relevant items, improving user engagement.
Example: Inventory ManagementMachine learning algorithms predict demand patterns, optimizing stock levels and reducing waste. Retailers can automate inventory replenishment processes, ensuring products are available when needed and minimizing excess inventory costs.
Innovative Solutions
Machine learning is driving innovation beyond traditional applications, enabling new possibilities and improving user experiences.
Natural Language Processing (NLP) and Computer Vision
Natural language processing (NLP) and computer vision are two areas where machine learning excels, enabling machines to understand and interpret human language and visual data.
Example: Chatbots and Virtual AssistantsNLP-powered chatbots provide instant customer support, answering queries and assisting users with tasks. Virtual assistants like Siri and Alexa leverage NLP to understand voice commands and perform actions, making everyday interactions more seamless.
Example: Image RecognitionComputer vision algorithms analyze images and videos to identify objects, people, and scenes. Applications range from facial recognition for security purposes to automated image tagging on social media platforms.
Event Registry: Unleashing Media Intelligence
At Event Registry, machine learning is at the core of organizing global news into coherent events. Our platform uses ML algorithms to analyze news articles, detect patterns, and group related content, providing users with comprehensive insights into trending topics and emerging issues. By turning vast amounts of news data into actionable intelligence, Event Registry empowers organizations to make informed decisions and stay ahead in a rapidly changing world. If you want to know how Event Registry transforms global news into actionable insights, or how our News API can provide tailored information, click here.
Challenges and Opportunities in Machine Learning
Overcoming Data Challenges
While machine learning offers immense potential, it also faces challenges, particularly related to data quality and diversity. Training robust ML models requires large, diverse datasets that accurately represent real-world scenarios. Ensuring data privacy and ethical usage is equally important to maintain trust and compliance.
Importance of Data Quality and Diversity
High-quality data is crucial for training accurate and reliable machine learning models. Datasets should be representative, diverse, and free from bias to ensure models generalize well to different scenarios. Addressing data quality issues and collecting diverse data sources are essential steps in building robust ML systems.
Privacy Concerns and Data Ethics
As machine learning relies on large datasets, privacy concerns and data ethics become paramount. Organizations must handle sensitive data responsibly, ensuring compliance with regulations and protecting user privacy. Implementing data anonymization, encryption, and ethical guidelines is crucial to maintaining public trust.
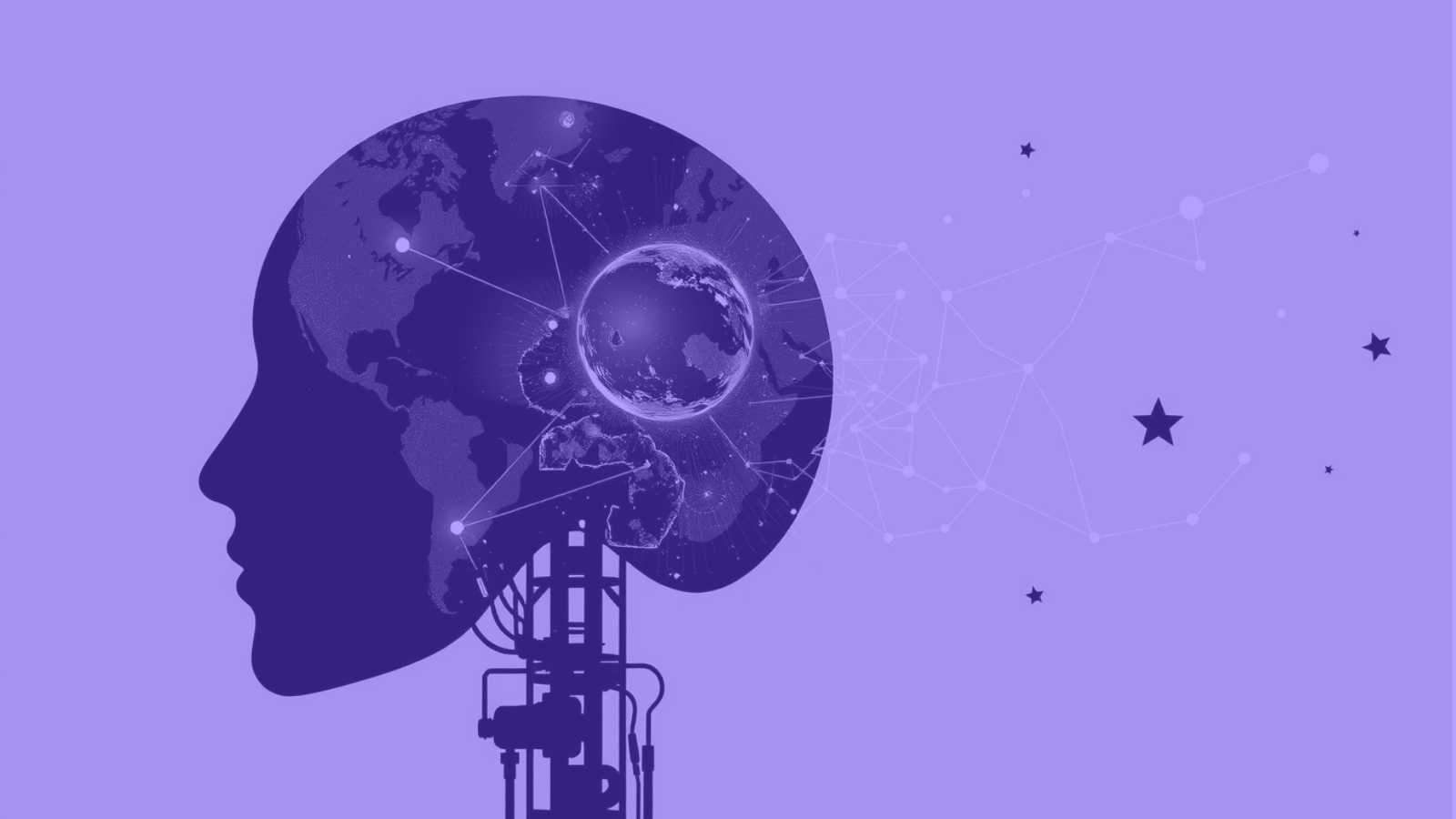
Future Directions
The future of machine learning holds exciting possibilities for addressing complex global issues:
- Climate Change: Machine learning can be leveraged to analyze climate data, model environmental trends, and develop sustainable solutions for mitigating climate change.
- Healthcare Access: ML algorithms can improve healthcare access by enabling remote diagnostics and telemedicine, bringing quality care to underserved communities.
Ongoing research and development in machine learning are focused on enhancing algorithmic capabilities, increasing model interpretability, and addressing ethical concerns, paving the way for even more transformative applications.
Conclusion
Machine learning isn't just a buzzword—it's the driving force behind some of the most exciting changes in our daily lives. From healthcare and finance to retail and beyond, ML is reshaping how we live, work, and interact with technology. The possibilities for personalization, efficiency, and smarter decision-making are limitless, but we also need to address the challenges to ensure its impact remains positive and beneficial.
Curious about what makes AI tick? Up next in our "AI Demystified" series are neural networks & deep learning, the brain power behind AI Magic. We'll dive into the fascinating world of neural networks—how they learn, how they think, and why they're the real brainpower behind AI's capabilities. Get ready for a journey into the depths of AI that will be equal parts enlightening and exciting!